Artificial Intelligence (AI) has revolutionized the healthcare industry, from diagnosing diseases to developing treatments.
Radiology, a medical specialty that uses medical imaging technology to diagnose and treat diseases, has not been left out.
AI-powered medical imaging technology has been developed and is rapidly gaining popularity in radiology. However, the big question is, will AI replace radiology?
This article explores the possible impacts and recommended skills that one should learn as a radiologist to overcome the challenges of AI, its limitations, and the future of radiology in the era of AI.
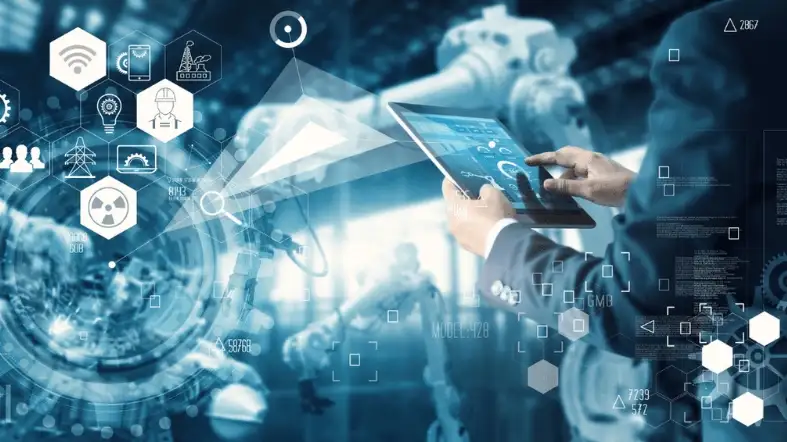
Will Ai Replace Radiology?
No, AI will not replace radiology, but it can assist radiologists in accurately diagnosing medical images.
Although the AI was able to diagnose some radiographs that were misinterpreted by human radiologists correctly, it still requires more training to reach the same level of performance as an average radiologist.
Furthermore, there are cases that the AI cannot interpret, requiring a human radiologist’s expertise.
What Challenges Are Faced In The Adoption Of AI In Radiology?
Insufficient and Inadequate Data Sets
The lack of adequate and well-curated datasets for training and testing AI algorithms in radiology is a major obstacle to its adoption.
The dataset needs to be diverse, comprehensive, and of high quality to ensure that the AI system is accurately trained.
This requires a significant amount of effort and resources and the cooperation of multiple institutions to share their data.
The Complexity of Demands and Staffing Shortages
Radiologists already have a high workload, and adding more demands to their already complex workflow can exacerbate burnout and staffing shortages.
Radiologists must manage the workload and the requirements for characterizing image datasets, which can further strain their resources and time.
Regulatory and Legal Issues
Another challenge in AI adoption in radiology is regulatory and legal issues.
Medical imaging is a heavily regulated industry, and AI algorithms must comply with various laws and regulations to ensure patient safety and privacy.
There are also concerns about liability and malpractice if an AI algorithm makes an incorrect diagnosis or misses a critical finding.
Radiologists and healthcare organizations must navigate these legal and regulatory challenges to ensure that AI is used effectively and safely in radiology.
Integration with Clinical Workflow
Integrating AI algorithms into clinical workflows is another significant challenge in AI adoption in radiology.
Radiologists and healthcare organizations must ensure that AI algorithms are seamlessly integrated into existing workflows to maximize efficiency and minimize disruptions.
Furthermore, AI algorithms must be easy to use and interpret, and radiologists must be trained to understand and trust the results.
This requires collaboration between radiologists, IT specialists, and other stakeholders to ensure that AI is integrated effectively and efficiently into clinical workflows.
Cost and Return on Investment
Expenditure and return on investment are important factors in adopting AI in radiology.
AI technology can be expensive to implement and maintain, and there may be limited financial incentives for radiologists and healthcare organizations to adopt AI.
However, the potential benefits of AI in radiology, such as increased accuracy, efficiency, and reduced costs, may outweigh the initial investment in AI technology.
Radiologists and healthcare organizations must carefully evaluate the costs and benefits of AI adoption to ensure that it is a viable and sustainable solution for improving patient care.
Clinician Input and Data Bottlenecks
Clinician input and data bottlenecks are two critical factors that can make AI technology less helpful than promised.
Clinicians must be involved in developing and implementing AI technology to ensure that it is useful and relevant to their needs.
Data quality and availability are also essential factors that can affect the accuracy and usefulness of AI in radiology.
How Can Ai Accurately Interpret Medical Images In Radiology?
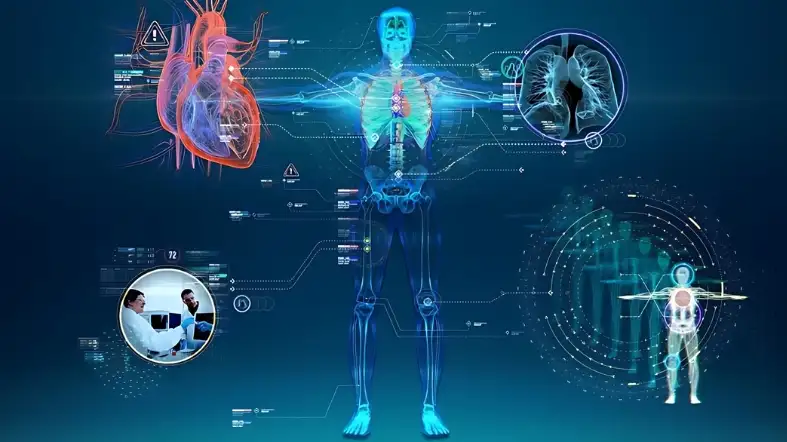
Image Acquisition
The first step in any medical imaging study is image acquisition. The patient undergoes a scan, and the images are saved in digital format.
Radiologists then analyze the images to diagnose any abnormalities. In the case of AI, the images are fed into an algorithm that can analyze them in a matter of seconds.
Preprocessing
Before the images are analyzed by the AI algorithm, they need to be preprocessed.
Preprocessing involves removing any artifacts or noise from the image, which can interfere with the AI algorithm’s ability to analyze the image accurately.
Preprocessing may also involve scaling or resizing the image to improve processing speed.
Training the Algorithm
To accurately analyze medical images, AI algorithms need to be trained on large datasets of labeled images.
These datasets are used to teach the algorithm how to recognize patterns in the images and differentiate between normal and abnormal images.
The more data the algorithm is trained on, the more accurate it becomes.
Analyzing the Image
Once the AI algorithm has been trained, it can be used to analyze medical images.
The algorithm uses deep learning techniques to analyze the image and identify any abnormalities.
It can also provide a probability score for the abnormality, indicating the likelihood of it being a disease.
Integrating AI into Clinical Practice
Integrating AI into clinical practice involves ensuring that the AI algorithm is reliable and accurate.
This is done by comparing the results of the algorithm with those of human radiologists.
If the AI algorithm’s results are consistent with those of human radiologists, it can be integrated into clinical practice.
What Are The Potential Benefits Of Using AI In Radiology?
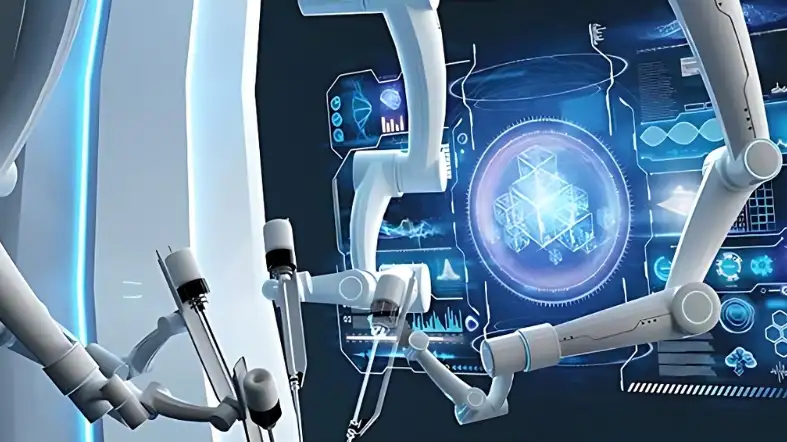
Quicker And More Precise Diagnosis
The ability to identify medical issues more quickly and correctly is one of the most important advantages of employing AI in radiology.
Radiologists may now more easily spot anomalies that can be hard to see with the naked eye thanks to AI algorithms that can quickly and efficiently evaluate enormous volumes of medical imaging data.
For instance, AI may assist radiologists in locating microscopic nodules or microcalcifications that could be signs of breast cancer in its early stages.
Better Patient Results
Patients are more likely to get prompt and effective treatment when radiologists can detect medical disorders more precisely and quickly.
Better health outcomes, cheaper healthcare expenditures, and a decrease in death rates may result from this.
Radiologists may diagnose patients more quickly by using AI to assist them in spotting patterns in medical pictures that can point to a specific illness or condition.
Plans for Individualized Treatment
By examining their medical photos and spotting patterns that may suggest a particular sickness or condition, AI may assist radiologists in customizing treatment programs to meet the requirements of individual patients.
Radiologists may then develop individualized treatment regimens for individuals that take into consideration their specific medical histories and present-day health conditions.
For instance, depending on the patient’s medical pictures and other characteristics,
AI algorithms may assist radiologists in determining the optimal treatment choice for a certain form of cancer.
Radiologists’ Workloads Are Lessened
In a single day, radiologists may have to examine hundreds or even thousands of medical pictures.
This may take a lot of time and energy and result in mistakes and exhaustion.
By automating some elements of their work, such as spotting anomalies in medical pictures or flagging areas of interest that need additional inspection, AI may reduce the burden for radiologists.
Radiologists may therefore be able to concentrate on other aspects of patient care, such as conferring with other medical specialists and creating treatment regimens.
Enhanced Healthcare Access
Patients may have to wait weeks or even months for a radiologist to evaluate their medical photos in places where access to healthcare is scarce.
By automating some of the radiologist’s work, AI may assist in solving this problem and enable patients to obtain a diagnosis and treatment plan much more quickly than in the past.
This may be very helpful in remote locations where there may be a scarcity of medical experts.
What Are The Limitations Of Using AI In Radiology?
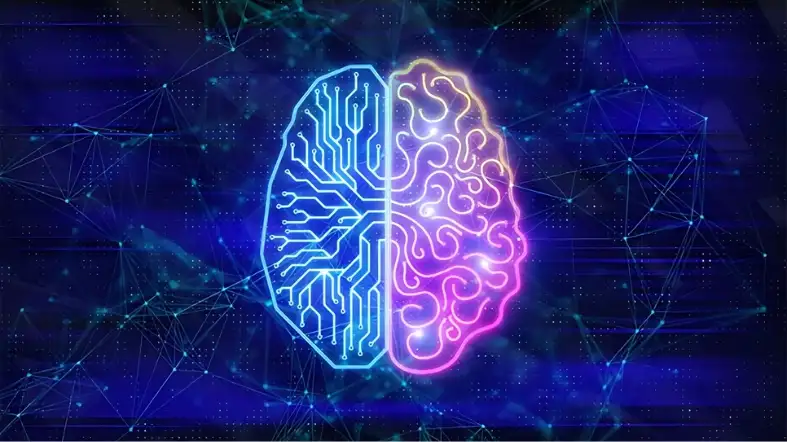
Lack of Sufficient Data
Lack of enough data is one of the main drawbacks of employing AI in radiology.
To learn and produce reliable predictions, AI systems need a lot of data. The AI system may not be able to perform as well as a human radiologist without sufficient data.
For instance, the AI system may not be able to identify a condition properly if it has only seen a small number of instances of it.
Bias in Data
The possibility of data bias is another restriction of AI in radiology. The quality of AI algorithms depends on the data they are trained on.
The algorithm may provide biased results if the data used to train it is biased.
For instance, if a certain demographic group dominates the data used to train an AI system, the algorithm may not be able to generalize to other groups correctly.
Inability to Explain Results
Although AI algorithms are quite good at identifying medical diseases, they often cannot articulate how they came to their results.
The “black box” issue, which refers to this inability to explain findings, may have a big impact on radiology.
For example, if an AI program identifies a tumour in a patient’s scan, it may not be able to justify its decision.
Difficulty in Integrating with Current Systems
It might be quite difficult to integrate AI technologies with the present radiology systems.
AI algorithms must be compatible with the large range of imaging methods and tools used by radiology departments.
Additionally, integrating AI systems with current radiology operations might be difficult and time-consuming.
Ethical Concerns
Ethical questions are raised by the use of AI in radiography. For instance, a false negative result from an AI system may cause a patient’s diagnosis and treatment to be delayed.
Patients could not believe the outcomes if the AI system is opaque in its decision-making process.
FAQs
Can Radiologists Be Entirely Replaced By AI?
Despite encouraging outcomes, it is doubtful that AI will completely replace radiologists in their profession.
AI is presently being developed as a tool to supplement, not completely replace, radiologists’ special clinical skills.
What Are Some Current Applications Of AI In Radiology?
In radiology, artificial intelligence (AI) is being utilized to facilitate picture identification and processing tasks, including tumor size estimation and abnormality detection.
Additionally, it is utilized to help with process efficiency and optimization as well as picture categorization and segmentation.
Can AI Make Radiology Diagnostics More Accurate?
By assisting radiologists in identifying anomalies that would have been overlooked or difficult to identify on a conventional scan, AI has the potential to increase the accuracy of radiology diagnosis.
But before it can be widely used, AI needs further testing and validation since it is still in the research stage.
How Can AI Serve Both Patients And Radiology Practices?
AI may help radiology practices and patients by cutting down on expenses and time spent on diagnosis, increasing the precision of diagnoses, and letting radiologists concentrate on more complicated situations that call for their expertise.
How Should Radiologists Be Ready To Incorporate AI Into Their Work?
By keeping up with the most recent advancements in AI and actively seeking out chances to learn how to utilize AI tools successfully, radiologists may be ready for the incorporation of AI into their practice.
Additionally, they may concentrate on honing their clinical skills and working with AI technologies to enhance patient outcomes.
Conclusion
Compared to conventional radiography, AI-powered medical imaging technology offers various benefits, including improved productivity, accuracy, and diagnostic capabilities.
The absence of emotional intelligence and reliance on high-quality data are two drawbacks of AI in radiology.
It is very early in the development of AI’s use in radiology; thus, it is difficult to predict what the future may bring.
While AI can potentially replace certain elements of radiology, radiologists are unlikely to be totally replaced by AI any time soon.